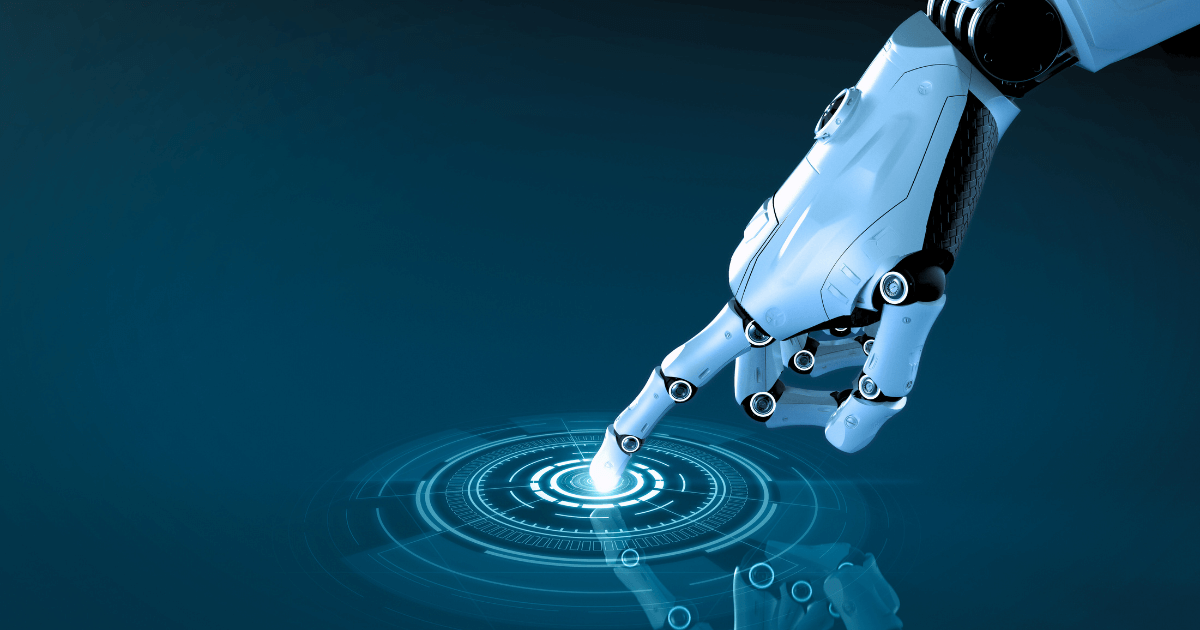
In Perifery’s paper on the “Evolution of Media Archiving”, we looked at common principles that all data archivers should build their archive strategies around.
Of the 10 principles in the paper, one dealt with the usage of AI in archiving. Arguably it could have been more, as AI has become ubiquitous in our thinking and lexicon as we strategize for the future. So, let’s look at how, in today’s data-driven world, the integration of Artificial Intelligence (AI) and Machine Learning (ML) into data archiving is revolutionizing how we manage, search, and utilize vast amounts of information.
The transformation isn't just a technological upgrade; it's a paradigm shift that enables unprecedented efficiencies and capabilities.
Intelligent Data Classification
Traditional data classification methods are often labor-intensive and prone to errors. AI changes this by analyzing and classifying archived data automatically. By identifying patterns, keywords, and content types, AI systems can categorize data with incredible accuracy. For instance, in media archives, AI can analyze video content to generate descriptive tags, annotations, and even create summaries or transcripts. This not only streamlines the archiving process but also ensures that data is categorized in a way that enhances future retrieval and usability.
Automated Metadata Generation
Metadata is crucial for understanding and accessing archived content but generating it manually can be cumbersome. AI excels at this task by automatically generating descriptive metadata such as tags and keywords. With video, AI can go a step further by employing facial recognition, object recognition, speech-to-text, and semantic scene recognition (e.g., identifying "car chases" or "love scenes"). This rich metadata layer transforms raw data into a well-annotated resource, making it significantly easier to navigate and utilize.
Enhanced Search and Retrieval
Searching through archives can be like finding a needle in a haystack. AI-powered search algorithms improve both the accuracy and speed of this process. Natural Language Processing (NLP) and ML techniques enable advanced search capabilities, such as semantic search or context-based retrieval. For example, semantic video searching allows users to search for specific scenes within videos, like "car chases," which can then be combined with other search parameters to pinpoint exact moments of interest. This makes the archive not just a repository of data, but a highly interactive and accessible tool.
Content-Based Recommendation
Discoverability within an archive is greatly enhanced by AI's ability to provide personalized recommendations. By analyzing the content of archived data, AI can suggest related or relevant content based on user preferences or viewing history. This capability transforms static archives into dynamic platforms for serendipitous exploration, increasing user engagement and the archive's overall value.
Video Analytics
AI's advanced video analytics capabilities unlock valuable insights from video content. AI can track objects, analyze crowds, assess sentiments, and detect events within videos. This turns archived videos into rich sources of information that can be leveraged for various purposes, from security and surveillance to marketing and user behavior analysis.
Video Restoration
Preserving the visual integrity of archived videos is paramount, and AI can help by automatically restoring and enhancing video quality. By reducing noise, stabilizing shaky footage, and improving resolution, AI ensures that archived videos are not only preserved but also optimized for future viewing. This enhances the longevity and usability of video archives.
AIOps: Enhancing IT Management of Archives
Beyond data management, AI Operations (AIOps) play a crucial role in the IT side of archiving. Here are three key enhancements:
- Data Deduplication
AIOps techniques can identify and eliminate duplicate data within the archive. This reduces storage costs and improves efficiency, ensuring that each piece of data is unique and valuable. - Predictive Storage Management
By analyzing usage patterns and access history, AIOps can predict future storage needs. This allows for optimized storage allocation, backup schedules, and migration strategies, ensuring that the archive grows in a manageable and cost-effective manner. - Intelligent Data Retention Policies
AIOps can evaluate the value, relevance, and legal requirements of archived data to determine appropriate retention and disposal policies. This ensures compliance and keeps the archive lean and relevant.
Data Gravity and Application Management
A modern archiving solution must also adhere to the principle of "data gravity," which suggests that as data accumulates, it pulls applications and services towards it rather than the other way around. Given the massive size of media libraries, often spanning hundreds of petabytes, moving data for processing is impractical and resource intensive. Instead, applications should come to the data.
This principle implies that a good data manager must also be an adept application manager, capable of deploying applications to the data. The archive should be able to run applications securely, scalably, and efficiently where the data resides. This involves a versatile approach that spans edge, on-premise, and cloud environments, ensuring that data processing is always performed in the most optimal location.
The integration of AI into data archiving represents a leap forward in how we manage, access, and utilize our data. From intelligent classification and automated metadata generation to enhanced search, personalized recommendations, advanced analytics, and video restoration, AI transforms static archives into dynamic, accessible, and valuable resources.
Coupled with AIOps for efficient IT management and the principle of data gravity for optimal application deployment, the future of data archiving is not just about storage but about intelligent, efficient, and effective data management. This shift not only enhances the value of the archives but also paves the way for new opportunities in data utilization and monetization.
To learn how Perifery is harnessing the power of AI to enable media workflows to better ingest, preserve, and distribute assets, and discover long-forgotten content for reuse and monetization, explore our AI+ data sheet.