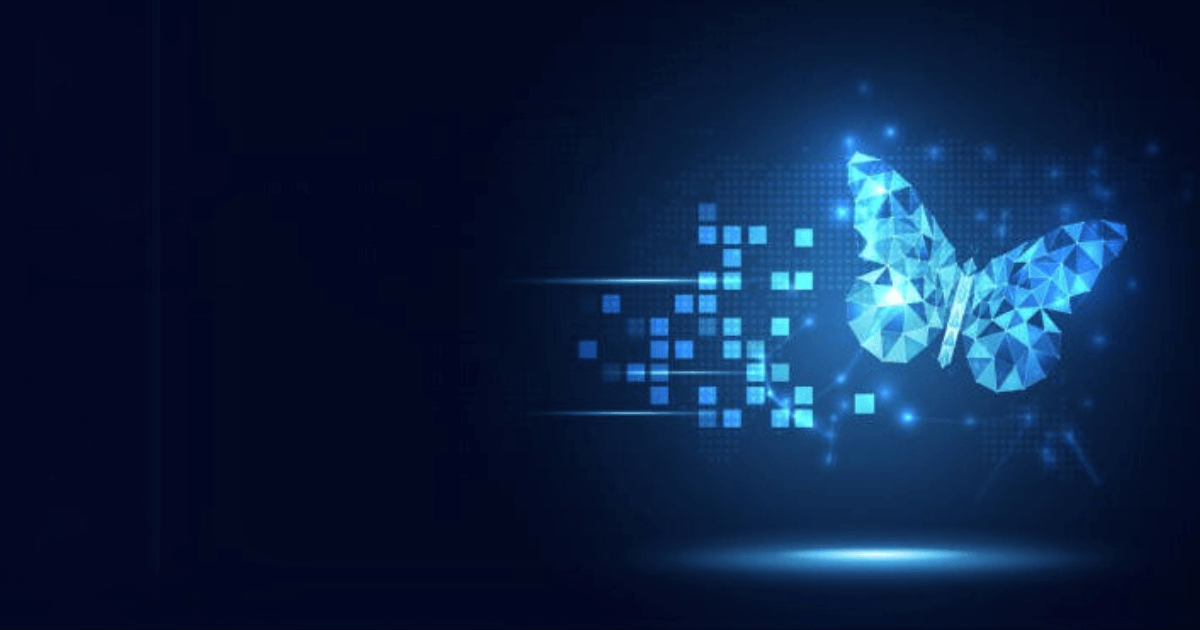
Key Takeaways
- AI-Driven Metadata Generation: Integrating modern AI to automate metadata generation eliminates the mundane task for humans while improving speed, accuracy and breadth of information captured.
- Expanded Search Capabilities: Using automated metadata generation that leverages facial and object recognition, transcription, and translation provides versatile and robust search opportunities. For example, metadata can be translated into multiple languages so end users can search in their native language.
- Open-Source Flexibility: Using open-source AI models future-proofs workflows by making updates simpler. It is wise to avoid proprietary models as they will become quickly outdated.
- Strategic Partnerships: Choose vendors that are positioned to help you navigate the evolving landscape of AI workflows and active archives as both are crucial for long-term success in the M&E industry.
There’s good reason to get excited about using artificial intelligence (AI) to generate detailed, accurate, meaningful metadata for your media assets. For those who have had metadata entry added to their long list of tasks, it means time saved and the ability to focus on the critical strategic and creative aspects of their roles. For those managing M&E archives, it brings innovative capabilities that streamline discovery and access to valuable content for reuse and monetization.
You may be asking, “isn’t this just the same old story about metadata?” to which I’d boldly answer that no—it isn’t the same story because a new and exciting chapter is underway. This long-awaited chapter to the story brings the metamorphosis of metadata a step nearer to completion with a maturation milestone that is transforming and modernizing the face of media asset management for the M&E industry.
The History of Metadata
Metadata has a storied history that began in the world of Library Science in the mid 1800s. Small cards were designed for each book to include what we now call metadata— author, subject. title, date of publication and a location code (e.g., Dewey Decimal System). This painstaking process enabled librarians and library users to easily find books. It wasn’t until the 1980s that libraries migrated away from card catalogs to using digital databases.
In the 130 or so years from the development of the card catalog and the digitization of library catalogs, what might be considered the first description of metadata came from MIT’s Center for International Studies experts David Griffel and Stuart McIntosh in 1967.
“…we have statements in an object language about subject descriptions of data and token codes for the data. We also have statements in a meta language describing the data relationships and transformations, and ought/is relations between norm and data.”
However, it wasn’t until 1983 that the term “metadata” was coined, formed by combining the Latin term “meta” which means “transcending” and data (a term first used in 1646), which means “factual information.” Sir Tim Berners-Lee, acknowledged as the inventor of the World Wide Web, noted that the phrase “machine understandable” is key in his definition. “Metadata is machine understandable information about web resources or other things.
The Evolution of Metadata
Fast forward to the year 2011. Jason Scott (in a blog post) proclaims:
“Metadata, you see, is really a love note – it might be to yourself, but in fact it’s a love note to the person after you, or the machine after you, where you’ve saved someone that amount of time to find something by telling them what this thing is.”
System-generated metadata has long been valuable, and it has received a lot of attention over the past dozen years or so. However, what wasn’t system generated was often being added manually at great expense to organizations and to the chagrin of those tasked with performing such a mundane task.
The Metamorphosis of Metadata
Now, in 2024, the options for generating metadata with AI could be compared to the metamorphosis of a living organism, with a speed and scale that is almost incomprehensible. The era of AI-driven insights for metadata and the effects on asset searchability and discovery are in full bloom.
Did you know that there are over a million powerful, open-source AI models now available? Advanced facial recognition, object recognition, transcriptions that include context, translation, and the list goes on and on. With this automatically generated metadata now being paired with advanced search capabilities, content management and search as we know it is quickly becoming a relic of the past.
How does this work? Workflows can be designed to use powerful AI models that are trained to perform specific tasks. For example, you can have a text transcript created from the audio track of a video and then analyze the text to determine topics, content, and specific words, speech patterns, or meanings. You can also run facial and object recognition models to identify who and what is portrayed in the video. Instead of taking days or weeks when done by a human, this can be done more thoroughly and with extreme accuracy by an AI model in a highly time-efficient manner. For example, to process a 2-hour video for metadata could take just a few seconds up to a few hours, depending on the complexity of the task and the speed of the hardware. While it is difficult to pinpoint exactly how much time it may take for a specific use case, it dramatically reduces the time and effort required for metadata creation/tagging when compared to traditional manual methods. Of course, AI is constantly evolving as new models are being developed, which will further speed up processing and even further improve the quality of metadata enrichment.
Perifery: Here to Help You Succeed for the Long Haul
To keep up with the ever-changing landscape of AI technology, you need to use solutions that will be flexible for the long haul. You may have noticed the mention of open-source AI models in the previous section and might be wondering why we advocate the use of open source. It is all about futureproofing your workflows. If you use a specific vendor’s proprietary AI model, it can quickly become outdated. When you use open-source AI models, updates can easily be made when new models are released. This is a critical consideration when you are choosing your path to using AI models.
Our teams at Perifery have long been focused on helping organizations succeed in both the short and long term with our smart storage platforms. In fact, content searchability and discoverability is in the DNA of our highly intelligent object-based storage solutions. Object-based storage is ideal for protecting media assets and building scalable archives, giving you a pool of searchable assets, which is why Object Matrix and Swarm have been successfully used in the M&E industry for the past two decades. Perifery was established as a division of DataCore (the leader in software-defined storage) to bring the M&E industry the technology solutions that content-rich organizations need to be successful now and in the future. From our perspective, the use of AI is a natural extension of the paradigm of object-based storage.
We understand how crucial it is to use vendors that understand the open-source AI landscape as well as the intricacies of nearline and active archive storage. Those vendors, who we are proud to call our partners, are best qualified to help you determine the right option to conquer your specific challenges. If you need help assessing the best AI tools for your metadata generation and other workflows or with building a smart storage pool to manage your media assets, contact us. We and our partners would be happy to help!